SAVING AND LOADING WEIGHTS https://pytorch-lightning.readthedocs.io/en/latest/common/weights_loading.html Saving and loading weights — PyTorch Lightning 1.5.0dev documentation Saving and loading weights Lightning automates saving and loading checkpoints. Checkpoints capture the exact value of all parameters used by a model. Checkpointing your training allows you to resume a training process in c..
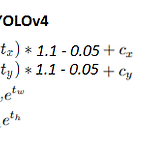
bounding box의 위치와 너비, 높이를 regression 하기 위한 수식이 각각 다르다. PyTorch 로 구현하고자 한다면 수식을 잘 보고 구현해야함 https://alexeyab84.medium.com/scaled-yolo-v4-is-the-best-neural-network-for-object-detection-on-ms-coco-dataset-3 bx와 by는 YOLOv4 페이퍼에서 언급됐듯이 grid sensitivity 를 고려하여 수정된 것인데... bw와 bh가 왜 저렇게 바뀐지 이유는 못찾았다. YOLOv5 도 Scaled-YOLOv4 의 수식을 따라가는듯하다. 알고보니 Scaled-YOLOv4 가 YOLOv5를 따른 것... https://github.com/ultralytic..
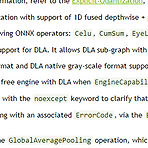
PyTorch 로 구현한 모델에 torch.gather 가 포함된 경우 ONNX 변환 시 ONNX 모델에 GatherElements 연산이 포함될 수 있다. onnx-tensorrt 프로젝트내에 Supported Operators에 관한 문서를 보면 GatherElements를 지원하나, TensorRT 버전에 따라 지원이 될 수도 있고 안될수도 있다. 적어도 7.2.1.6 버전에서는 지원이 안되는 것을 확인하였다. (ONNX2TensorRT 과정은 내가 맡은 부분이 아니라 확실하진 않다...) https://github.com/onnx/onnx-tensorrt/blob/master/docs/operators.md onnx/onnx-tensorrt ONNX-TensorRT: TensorRT backend..
기존에 Loss를 항상 float32타입으로 계산하도록 코드를 짰었다. 이유는 AMP를 쓸때 Loss를 float16타입으로 계산하면 정밀도가 떨어지고 오버플로우 문제가 발생할걸로 예상했기 때문... 근데 이렇게 하니까 AMP 사용해서 학습시키니 모델 성능이 크게 저하됨(VOC 데이터셋 기준 mAP 가 2.0 정도 드랍됨, 모델은 CenterNet-ResNet18 기준) 이부분을 모델 아웃풋 텐서의 타입으로 맞춰주니 성능 저하 없고 오히려 성능이 향상됨 아래 프로젝트 개발하다 해당 문제를 발견하게됨 https://github.com/developer0hye/Simple-CenterNet developer0hye/Simple-CenterNet PyTorch Implementation of CenterNet..
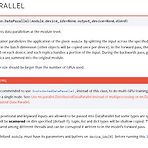
기존에 Single node, multiple GPUs System(그냥 PC 1대에 GPU 여러대 꽂힌 피시로 생각, pytorch 공식 문서에서 이렇게 표기했길래 따라 씀) 에서 multiple gpu 를 활용함에 있어 DataParallel 모듈을 활용했다. 그런데, 쓰다보니 GPU 메모리 불균형 문제가 너무 심해서 메모리가 쏠리는 한 GPU 가 터지지 않게 해당 GPU의 메모리 사용량에 배치사이즈를 맞추다보니 다수개의 GPU를 제대로 활용하지 못했었다. 이와 관련해서는 아래의 포스트에서도 언급되어 있다. https://medium.com/daangn/pytorch-multi-gpu-%ED%95%99%EC%8A%B5-%EC%A0%9C%EB%8C%80%EB%A1%9C-%ED%95%98%EA%B8%B0..
import timm model_name = 'your_model' model = timm.create_model(model_name, pretrained=True, features_only=True).cuda() model.feature_info.channels() model.feature_info.channels() 값 확인해보면 스테이지별 채널 수를 확인할 수 있음.
https://pytorch.org/docs/stable/notes/amp_examples.html#id6 Automatic Mixed Precision examples — PyTorch 1.9.0 documentation Shortcuts pytorch.org scaler = GradScaler() for epoch in epochs: for i, (input, target) in enumerate(data): with autocast(): output = model(input) loss = loss_fn(output, target) loss = loss / iters_to_accumulate # Accumulates scaled gradients. scaler.scale(loss).backward()..
PyTorch 모델을 Tensorrt로 변환하기 위해 많은 방법이 있겠지만, 그중에 하나는 PyTorch -> Onnx -> TensorRT 로 변환을 하는 것이다. 위 과정이 매끄럽게 진행 되기 위해선 나의 PyTorch 모델이 Onnx 에서 지원하는 연산들을 통해 변환되는지부터 확인해야 하고, Onnx 연산들이 또 Tensorrt에서 지원되는지 확인해야한다. PyTorch to Onnx https://pytorch.org/docs/stable/onnx.html#supported-operators torch.onnx — PyTorch 1.8.1 documentation Shortcuts pytorch.org 위 링크에서는 PyTorch to Onnx 과정에서 공식적으로 지원되는 연산들을 확인할 수 있다..
github.com/ultralytics/yolov3/issues/1098 Memory Friendly Mish() Activation · Issue #1098 · ultralytics/yolov3 Hello, I tried yolov4.cfg on 2080Ti with input size 448*448, the training runs out of cuda memory even with batch size = 8 Have you tried the largest batch size value which won't break the cuda... github.com Mish activiation function을 사용하면 메모리 사용량이 크게 증가하는 것으로 보인다. Single Gpu에서 학습 가능한 모..
- Total
- Today
- Yesterday
- ㅂ
- 파이참
- cosine
- 위상 정렬 알고리즘
- 가장 긴 증가하는 부분 수열
- 단축키
- 조합
- C++ Deploy
- 인공지능을 위한 선형대수
- Lowest Common Ancestor
- PyCharm
- FairMOT
- 백준 11053
- 백준 11437
- 백준
- 백트래킹
- 순열
- 이분탐색
- MOT
- LCA
- 백준 1766
- 자료구조
- 문제집
일 | 월 | 화 | 수 | 목 | 금 | 토 |
---|---|---|---|---|---|---|
1 | 2 | 3 | 4 | |||
5 | 6 | 7 | 8 | 9 | 10 | 11 |
12 | 13 | 14 | 15 | 16 | 17 | 18 |
19 | 20 | 21 | 22 | 23 | 24 | 25 |
26 | 27 | 28 | 29 | 30 | 31 |