티스토리 뷰
MobileNetv1은 대표적인 컨볼루셔널 레이어 기반의 경량(Lightweight) 네트워크입니다. Depthwise Convolution과 Pointwise Convolution을 적절히 사용함으로써 타 네트워크(vgg, googlenet등) 대비 크게 적은 파라미터수와 연산량으로 대등한 인식 성능을 보이며 세간의 관심을 끌었습니다.
논문 링크(Official)
MobileNets: Efficient Convolutional Neural Networks for Mobile Vision Applications
We present a class of efficient models called MobileNets for mobile and embedded vision applications. MobileNets are based on a streamlined architecture that uses depth-wise separable convolutions to build light weight deep neural networks. We introduce tw
arxiv.org
다음은 MobileNetv1 논문에 실린 MobileNetv1의 구조를 나타낸 테이블입니다. 여기서 Conv는 일반적인 Convolutional Layer를 의미하고, Conv dw는 Depthwise Convolutional Layer를 의미합니다. FC는 Fully Connected Layer를 의미합니다.
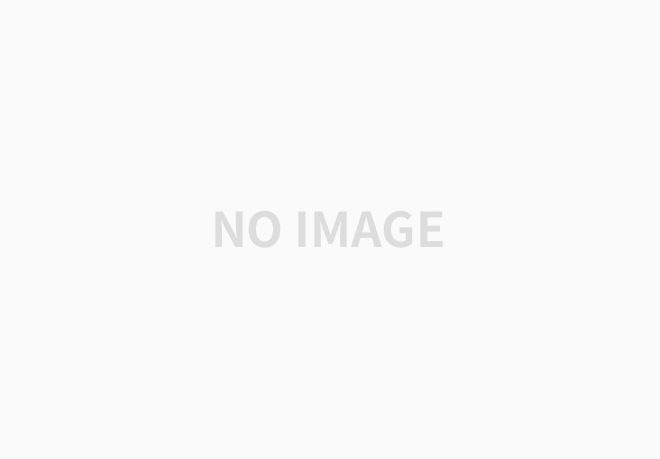
그런데 실제 MobileNetv1 코드를 분석해보면, 해당 테이블과 일치하지 않는 부분이 존재합니다. 아래의 코드를 참고해보시길 바랍니다.
텐서플로 기반 소스코드 링크(Official)
github.com/tensorflow/models/blob/master/research/slim/nets/mobilenet_v1.py
tensorflow/models
Models and examples built with TensorFlow. Contribute to tensorflow/models development by creating an account on GitHub.
github.com
파이토치 기반 소스코드 링크(Not Official)
아래 코드에서 conv_dw 함수는 위 테이블 상의 Conv dw와 대응되지 않으니 분석하시는데 주의하시길 바랍니다.
github.com/jmjeon94/MobileNet-Pytorch/blob/master/MobileNetV1.py
jmjeon94/MobileNet-Pytorch
Implementation of MobileNet V1, V2, V3. Contribute to jmjeon94/MobileNet-Pytorch development by creating an account on GitHub.
github.com
코드를 참고하여 테이블을 다시 작성해보겠습니다. 실제 구현과 차이가 있는 부분을 빨간색 글씨로 재표기 하였습니다.
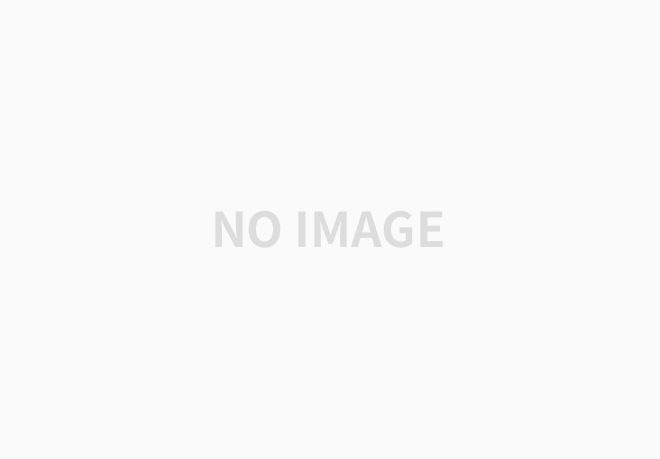
MobileNetv1을 분석하는데 도움이 되었기를 바랍니다.
'Deep Learning' 카테고리의 다른 글
Empirical study on label smoothing in neural networks (0) | 2021.02.27 |
---|---|
YOLOv3 구현을 위해 참고할 자료들 (0) | 2021.01.16 |
Windows 10 GPU Status 확인 (0) | 2020.12.23 |
MOT Dataset Annotation Format 정리 (9) | 2020.11.22 |
MOT20 Ground Truth Format (0) | 2020.11.17 |
- Total
- Today
- Yesterday
- LCA
- 위상 정렬 알고리즘
- FairMOT
- MOT
- 백트래킹
- C++ Deploy
- 단축키
- PyCharm
- 순열
- cosine
- 문제집
- 백준 11053
- Lowest Common Ancestor
- 파이참
- 자료구조
- 백준 11437
- 인공지능을 위한 선형대수
- 백준
- 조합
- ㅂ
- 가장 긴 증가하는 부분 수열
- 백준 1766
- 이분탐색
일 | 월 | 화 | 수 | 목 | 금 | 토 |
---|---|---|---|---|---|---|
1 | 2 | 3 | 4 | 5 | ||
6 | 7 | 8 | 9 | 10 | 11 | 12 |
13 | 14 | 15 | 16 | 17 | 18 | 19 |
20 | 21 | 22 | 23 | 24 | 25 | 26 |
27 | 28 | 29 | 30 |